- Use the 'Significance' section to build the reader's interest in the problem(s) our Specific Aims address.
- Use the 'Innovation' section to build the reader's interest in the methods we will use to achieve our aims.
- Use the 'Approach' section to convince the reader that we can accomplish our Aims.
- Use the initial 'Specific Aims' page to summarize all of the above, both as an introduction for the few readers who will read the rest of the proposal and as a stand-alone summary for everyone else.
- Home
- Angry by Choice
- Catalogue of Organisms
- Chinleana
- Doc Madhattan
- Games with Words
- Genomics, Medicine, and Pseudoscience
- History of Geology
- Moss Plants and More
- Pleiotropy
- Plektix
- RRResearch
- Skeptic Wonder
- The Culture of Chemistry
- The Curious Wavefunction
- The Phytophactor
- The View from a Microbiologist
- Variety of Life
Field of Science
-
Carbon Content in Dwarf Stars1 week ago in Doc Madhattan
-
What I Read 20243 weeks ago in Angry by Choice
-
I've moved to Substack. Come join me there.2 months ago in Genomics, Medicine, and Pseudoscience
-
-
-
The Site is Dead, Long Live the Site2 years ago in Catalogue of Organisms
-
The Site is Dead, Long Live the Site2 years ago in Variety of Life
-
-
-
-
Histological Evidence of Trauma in Dicynodont Tusks6 years ago in Chinleana
-
Posted: July 21, 2018 at 03:03PM6 years ago in Field Notes
-
Why doesn't all the GTA get taken up?6 years ago in RRResearch
-
-
Harnessing innate immunity to cure HIV8 years ago in Rule of 6ix
-
-
-
-
-
-
post doc job opportunity on ribosome biochemistry!10 years ago in Protein Evolution and Other Musings
-
Blogging Microbes- Communicating Microbiology to Netizens10 years ago in Memoirs of a Defective Brain
-
Re-Blog: June Was 6th Warmest Globally10 years ago in The View from a Microbiologist
-
-
-
The Lure of the Obscure? Guest Post by Frank Stahl12 years ago in Sex, Genes & Evolution
-
-
Lab Rat Moving House13 years ago in Life of a Lab Rat
-
Goodbye FoS, thanks for all the laughs13 years ago in Disease Prone
-
-
Slideshow of NASA's Stardust-NExT Mission Comet Tempel 1 Flyby14 years ago in The Large Picture Blog
-
in The Biology Files
Not your typical science blog, but an 'open science' research blog. Watch me fumbling my way towards understanding how and why bacteria take up DNA, and getting distracted by other cool questions.
PHS 398 gestalt
A paradigm shift for how I organize grant proposals?
Specific Aims
This comes first; it has a one-page limit. I think it must also serve as a Summary page, because I can't find any mention of a separate Summary in the Instructions.
- State concisely the goals of the proposed research and summarize the expected outcome(s), including the impact that the results of the proposed research will exert on the research field(s) involved.
- List succinctly the specific objectives of the research proposed, e.g., to test a stated hypothesis, create a novel design, solve a specific problem, challenge an existing paradigm or clinical practice, address a critical barrier to progress in the field, or develop new technology.
(a) Significance
- Explain the importance of the problem or critical barrier to progress in the field that the proposed project addresses.
- Explain how the proposed project will improve scientific knowledge, technical capability, and/or clinical practice in one or more broad fields.
- Describe how the concepts, methods, technologies, treatments, services, or preventative interventions that drive this field will be changed if the proposed aims are achieved.
(b) Innovation
- Explain how the application challenges and seeks to shift current research or clinical practice paradigms.
- Describe any novel theoretical concepts, approaches or methodologies, instrumentation or intervention(s) to be developed or used, and any advantage over existing methodologies, instrumentation or intervention(s).
- Explain any refinements, improvements, or new applications of theoretical concepts, approaches or methodologies, instrumentation or interventions.
C. Approach
- Describe the overall strategy, methodology, and analyses to be used to accomplish the specific aims of the project. Include how the data will be collected, analyzed, and interpreted as well as any resource sharing plans as appropriate.
- Discuss potential problems, alternative strategies, and benchmarks for success anticipated to achieve the aims.
- If the project is in the early stages of development, describe any strategy to establish feasibility, and address the management of any high risk aspects of the proposed work.
- Discuss the PD/PI's preliminary studies, data, and/or experience pertinent to this application.
I promised myself that I'd have a rough draft by the end of November, so I'd better get busy converting my outline into paragraphs.
Improved trait mapping?
First a paragraph of background. Different strains of H. influenzae differ dramatically in how well they can take up DNA and recombine it into their chromosome (their 'transformability'). Transformation frequencies range from 10^-2 to less than 10^-8. We think that finding out why will help us understand the role of DNA uptake and transformation in H. influenzae biology, and how natural selection acts on these phenotypes. Many other kinds of bacteria show similar strain-to-strain variation in transformability, so this understanding will probably apply to all transformation. The first step is identifying the genetic differences responsible for the poor transformability, but that's not so easy to do, especially if there's more than one difference in any one strain.
Step 1: The first step we planned is to incubate competent cells of the highly transformable lab strain with DNA from the other strain we're using, which transforms 1000-10000 times more poorly. We can either just pool all the cells from the experiment, or first enrich the pool for competent cells by selecting those that have acquired an antibiotic resistance allele from that DNA. We expect the poor-transformability allele or alleles from the donor cells (call them tfo- alleles) to be present in a small fraction (maybe 2%?) of the cells in this pool.
Step 2: The original plan was to then make the pooled cells competent again, and transform them with a purified DNA fragment carrying a second antibiotic resistance allele. The cells that had acquired tfo- alleles would be underrepresented among (or even absent from) the new transformants, and, when we did mega-sequencing of the DNA from these pooled second transformants, the responsible alleles would be similarly underrepresented or absent.

Step 2*: First, instead of selecting in Step 2 for cells that can transform well, we might be able to screen individual colonies from Step 1 and pool those that transform badly. We have a way to do this - a single colony is sucked up into a pipette tip, briefly resuspended in medium containing antibiotic-resistant DNA, and then put on an antibiotic agar plate. Lab-strain colonies that transform normally usually give a small number of colonies, and those that transform poorly don't give any. Pooling all the colonies that give no transformants (or all the colonies that fall below some other cutoff) should dramatically enrich for the tfo- alleles, and greatly increase the sensitivity of the sequencing analysis. Instead of looking for alleles whose recombination frequency is lower than expected, we'll be looking for spikes, and we can increase the height of the spikes by increasing the stringency of our cutoff.

Step 1* (the RA's suggestion): Instead of transforming the lab strain with the poorly-transforming strain in Step 1, we could do the reverse, using DNA from the lab strain and competent cells from the poorly transformable strain. Step 2 would be unchanged; we would make the pooled transformants competent and transform them with a second antibiotic-resistance marker, selecting directly for cells that have acquired this marker. This would give us a pool of cells that have acquired the alleles that make the lab strain much more transformable, and again we would identify these as spikes in the recombination frequency.

The other problem with this approach is that we'd need to first make the inverse recombination map (the 'inverse recombinome'?) for transformation of lab-strain DNA into the tfo- strain. This would take lots of sequencing, so it might be something we'd plan to defer until sequencing gets even cheaper.
I think we may want to present all of these approaches as alternatives, because we're proposing proof-of-concept work rather than the final answer. The first two are simpler and will work even on (best on?) strains that do not transform at all. The last will work very well on strains that do transform at a low frequency..
Still not done
One of the runs I thought to have hung has finished, and it gives me enough data to fix the graph I needed it for. But I'm not going to do any more work on this until my co-authors have ahd a chance to consider whether we want to include this figure (I like it).
Getting the US variation manuscript off my hands
Below I'm going to try to summarize the new data (new simulation runs) I've generated. Right now I can't even remember what the runs were for, and I haven't properly analyzed any of them.
A. One pair of runs were two runs with 10 kb genomes that were intended to split the load of a 20 kb genome run that had stalled (needed only as one datapoint on a graph). That run had used a very low mutation rate and I was trying to run it for a million cycles, but it had stalled after 1.87x10^5 cycles. Well, it kept running, but not posting any more data so eventually I aborted it. Splitting it into two 10 kb runds didn't help - both hung after 1.87 x 10^5 cycles. Now I've made two changes. First, I've modified the 'PRINT' commands so that updates to the output file won't be stuck in the cluster's buffer; this may be why updates to the output files were so infrequent (sometimes not for weeks!). Second, I've set these runs to go for only 150,000 cycles and to report the genome sequences when they finish. This will let me use their output sequences as inputs for new runs.
B. Another pair of runs were duplicates of previous runs used to illustrate the equilibrium. One run started with a random-sequence genome and got better, the other started with a genome seeded with many perfect uptake sequences and got worse. They converge on the same final score, as seen in the figure below.

C. And one run was to correct a mistake I'd made in a 5000 cycle run that used the Neisseria meningitidis DUS matrix to specify its uptake bias. I should have set the mutation parameters and the random sequence it started with to have a base composition of 0.51 G+C, but absentmindedly used the H. influenzae value of 0.38. I needed the sequence that this run would produce, because I wanted to use the sequence outputs of it and its H. influenzae USS matrix equivalent as inputs for another 5000 cycles of evolution. I got the sequence from the first run, and started the second pair of runs, but unfortunately the computer cluster I'm using suffered a hiccup and those runs aborted. So I'll queue them again right now. (Pause while I re-queue them...)
D. Then there were four runs that used tiny fragments - enough 50, 25 and 10 bp fragments to cover 50% of the 200 kb genome. Because the length of the recombining fragments sets the minimum spacing of uptake sequences in equilibrium genomes, we expect runs using shorter fragments to give higher scores. But because the fragment mutation rate is 100-fold higher than the genomic rate in our simulations, most of the unselected mutations in our simulated genomes come in by recombination, in the sequences flanking uptake sequences. This means that genomes that recombine 10 bp fragments get few mutations outside of their uptake sequences, so I also ran the 10 bp simulation with a 10-fold higher mutation rate. These runs haven't finished yet - in fact, most of them have hardly begun after 24 hrs. I think I'd better set up new versions that use the bias-reduction feature, and then run the outputs of these in runs with unrelenting bias. (Pause again...)
The rest of the new runs were to fill in an important gap in what we'd done. The last paragraph of the Introduction promised that we would find out what conditions were necessary for molecular drive to cause accumulation of uptake sequences. But we hadn't really done that - i.e. we hadn't made an effort to pin down conditions where uptake sequences don't accumulate. Instead we'd just illustrated all the conditions where they do.
E. So one series of runs tested the effects of using lower recombination cutoffs (used with the additive versions of the matrix) when the matrix was relatively weak. I had data showing that uptake sequences didn't accumulate if the cutoff was less than 0.5, but only for the strong version of the matrix. Now I know that the same is true for the weak version.
F. Another series tested very small amounts of recombination. The lowest recombination I'd tested in the runs I had already done was 0.5% of the genome recombined each cycle, which seemed like a sensible limit as this is only one 100 bp fragment in a 20 kb genome. But this still gave substantial accumulation of uptake sequences, so now I've tested one 100 bp fragment in 50 kb, 100 kb and 200 kb genomes. I was initially surprised that the scores weren't lower, but then remembered that these scores were for the whole genome, and needed to be corrected for the longer lengths. And now I've also remembered that these analyses need to started with seeded sequences as well as random sequences, because this is the rigorous way we're identifying equilibria. (Another pause while I set up these runs and queue them...)
G. The final set of runs looked at what happens when a single large fragment (2, 5 or 10 kb) recombines into a 200 kb genome each cycle. Because there would otherwise be little mutation at positions away from uptake sequences, these runs also had a 10-fold elevated genomic mutation rate. The output genome sequences do have more uptake sequences than the initial random sequences, but the effect is quite small, and the scores for these runs were not significantly different than those for the runs described in the paragraph above, where the fragments were only 100 bp. This is expected (not that I think it through) because the only difference between the runs is that this set's fragments bring in 2-10 kb of random mutations in the DNA flanking the uptake sequence.
(I was going to add some more figures, but...)
Too many figures!
But where's the microscope?
This is the optical tweezers setup I'll initially be working with. The microscope slide chamber is clamped to the light-coloured micromanipulator controls at the center, with a water-immersion objective lens on its right side and a light condenser on its left side. The little black and yellow tube at the back left is the infrared laser, and the tall silver strip beside it holds the photodetector that detects the laser light after it is bounced through mirrors and lenses, the slide chamber and condenser, and another mirror. The visible light source is out of view on the left, and the rightmost black thing is the visible-light camera which lets you see what you're doing, via the grey cable that connects it to a computer screen. Lying on the table in front of the camera is a slide chamber, left by one of the biophysics students who've been using this apparatus.
Preparing for optical tweezers work

Faculty position cover letter Do's and Don't's
Do: Explain what distinguishes your work on regulatory protein-of-the-month from everyone else's.
Do: Recheck the final text just before you submit it.

Don't: Say that you're applying because you really want to live here.
Don't: Say that you only found out about the position because your buddy showed you the ad.
Don't: List generic research 'skills' such as gel electrophoresis, cell culture, and Microsoft Excel.
Don't: Say that you're the ideal candidate.
Don't: Say that you will happily apply your specialized techniques to any research question (i.e. you don't care about the science, you just want to play with your toys).
Don't: Give a full history of every project you've ever worked on.
Don't: Fill four pages.
Don't: Talk about your passion for research (or any other feelings).
Don't: Say you're hardworking.
Dont: Delay publishing a first-author paper on your post-doc work until you're about to apply for faculty positions.
Blue screen logouts in Excel - Numbers is not the answer.
I've got a lot of data to analyze from all the simulations I've run, so I'm going to try using the Mac Numbers app instead. Whoa, doesn't look good. Here's what the default for a line graph produced:
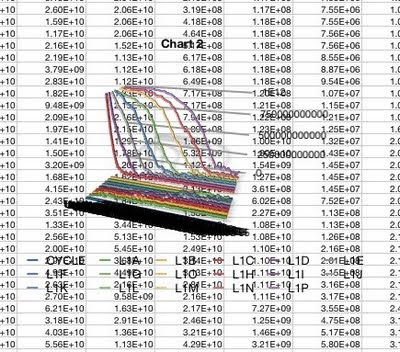
- The background is transparent.
- This is a 3-D graph.
- The lines are 3-d, like ropes.
- The lines have 3-D shadows.
- There are no visible axes.
- There is no scale.
- There are some numbers connected to some of the lines.
- The legend at the bottom seems to treat the X-axis values as Y-axis values.
Ah, the problems with the previous graph were partly that I had accidentally chosen 3-D. But my new graph is terrible too.
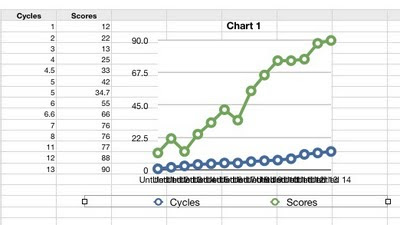
- The symbols are enormous and I can't find any way to change them or remove them.
- My X-axis values are being treated as Y-axis values - I don't know what it's using for the X-axis as the numbers are illegibly jammed together.
- My column headings (Cycles, Scores) are ignored.
Aha, changing to a scatter plot got it to use my Cycles data for the X-axis values. But now the lines connecting the points have disappeared and I see no way to get them back. And the new legend says that the Xs represent cycles, when they're really Scores. Instead moving the Cycles data over into the grey first column gave a semi-presentable line graph, but now it's not treating the Cycles values as numbers but as textual labels, even though I discovered how to tell it that they are numbers.
I'm afraid Numbers appears to be just a toy app, suitable for children's school projects but not for serious work. I would RTFM but I can't find anything sensible.
Uptake sequence stability
New! Improved! The NIH plan!
- Uptake bias (across the outer membrane)
- Translocation biases (across the inner membrane)
- Cytoplasmic biases (nucleases and protection)
- Strand-exchange biases (RecA-dependence)
- Mismatch repair biases
Now, where was I?
Genome BC's submission software...

Genome BC proposl angst
I'm alternating between thinking that our various drafts are written very bacly (so we should just abandon the proposal) and remembering that the science we propose is really dazzling. Back and forth and back and forth...
The horror!
- Signature page
- Participating Organizations Signatures ( ≠ signature page)
- Co-applicants
- Lay summary
- Summary
- Proposal (5 pages, plus 5 for references, figures etc.)
- Gantt chart (to be included with figures)
- SWOT analysis matrix and explanatory statement
- Strategic Outcomes (???)
- Project team
- Budget (Excel spreadsheet provided)
- Co-funding strategy
- Budget justification
- Documents supporting budget justification
- Suporting documents for co-funding
- List of researchers on the 'team' (just me and the post-doc?)
- Researcher profile for me
- Researcher profile for the post-doc
- List of collaborators and support
- Publications (we can attach five relevant pdfs)
- Certification forms form
- Biohazard certification form (we're supposed to get one especially for this proposal, but only if the project is approved)
limits set by sequencing errors
(*The limit becomes the square of the error rate, about 4 x 10^-6.)